7 TOP AI USE CASES IN LIFE SCIENCES in 2025 - AINGENS
The truth is that conventional medical research methods, disease diagnosis, and medication discovery are laborious, expensive, and prone to human error. However, AI is causing groundbreaking developments, speeding up innovation, cutting expenses, and increasing accuracy throughout the sector. AI accelerates research and improves drug safety, clinical trial efficiency, and therapeutic precision.
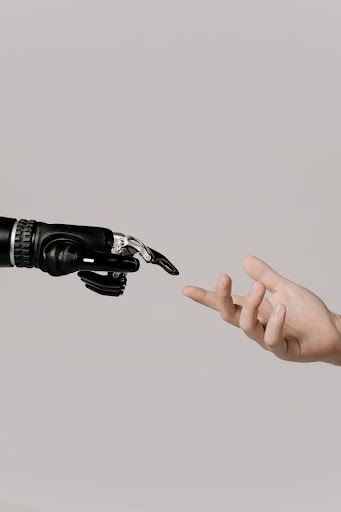
Have you ever wondered how artificial intelligence will change life sciences workflows in 2035?
Several AI use cases in life sciences are currently being explored, and many more will follow.
AI is propelling significant advancements in biotech, pharmaceuticals, and healthcare, from improving medical diagnostics to changing drug discovery.
The truth is that conventional medical research methods, disease diagnosis, and medication discovery are laborious, expensive, and prone to human error. However, AI is causing groundbreaking developments, speeding up innovation, cutting expenses, and increasing accuracy throughout the sector.
AI accelerates research and improves drug safety, clinical trial efficiency, and therapeutic precision.
This post will discuss the top seven AI use cases in life sciences influencing medical research and scientific advancement.
RELATED BLOG POSTS
- 13 MUST-KNOW AI TOOLS FOR LITERATURE REVIEWS [2025]
- 7 BEST MUST-HAVE AI TOOLS FOR MEDICAL STUDENTS [2025]
- MACg: THE BEST AI RESEARCH & WRITING ASSISTANT | AINGENS
- PROS AND CONS OF AI IN MEDICAL WRITING - AINGENS
Benefits of Using AI in Life Sciences
There are several benefits of AI use cases in life sciences, including:
Faster and more accurate research
AI’s ability to analyze massive data far surpasses human capabilities, enabling researchers to identify patterns and correlations that otherwise remain hidden.
AI algorithms can sift through countless research papers, clinical trials, and genetic databases, pinpointing key insights and potential breakthroughs. This speeds up the research process and enhances accuracy by minimizing human error and bias, leading to more reliable and reproducible results.
Enhanced diagnostics
AI is revolutionizing disease diagnosis through its ability to analyze medical images, such as X-rays, CT scans, and MRIs, with exceptional precision.
Deep learning models can detect subtle abnormalities that human observers may miss, leading to earlier and more accurate diagnoses.
AI also analyzes patient data, including electronic health records and genetic information, to identify risk factors and predict disease progression.
Personalized medicine
AI is a cornerstone of personalized medicine, enabling treatments to be tailored to individual patient needs.
AI algorithms can predict how patients will respond to different treatments by analyzing their genetic makeup, medical history, and lifestyle factors.
This allows clinicians to select the most effective therapies and minimize adverse drug reactions.
Improved efficiency and cost savings
AI automates numerous tasks in life sciences, including data entry and analysis. This reduces the workload on researchers and healthcare professionals, freeing their time for more complex and strategic tasks.
AI Use Cases in Life Sciences in 2025
As mentioned earlier, several AI use cases in life sciences range from drug discovery to personalized medicine.
However, we are only just scratching the surface of possibilities.
Here are some of the current and major AI use cases in life sciences today:
AI in drug discovery & development
Drug discovery and development is a lengthy, costly, and often inefficient process. AI is revolutionizing this field by accelerating various stages, such as:
- Target identification and validation
AI algorithms analyze vast amounts of data to identify potential drug targets. Machine learning models can predict the relevance of specific targets to diseases, prioritize them, and even suggest novel targets that might have been overlooked.
- Virtual screening and lead optimization
AI enables virtual screening of millions of compounds against potential drug targets, significantly reducing the number of compounds that need to be physically tested.
Generative AI models can even design novel molecular structures with desired properties, such as high binding affinity and low toxicity.
- Clinical trial design and analysis
AI can help design more efficient clinical trials by identifying suitable patient populations, optimizing dosing regimens, and predicting patient responses.
AI algorithms can analyze clinical trial data to identify patterns and insights that traditional statistical methods might miss, accelerating the evaluation of drug efficacy and safety.
- Drug repurposing
AI can analyze large datasets of existing drugs and disease data to find new uses for old drugs. This can significantly reduce the time and cost of drug development.
AI in disease diagnosis
AI is transforming disease diagnosis by enabling faster, more accurate, and less invasive methods. Here are some ways AI is revolutionizing disease diagnosis:
- Medical imaging analysis
Deep learning algorithms can analyze medical images, such as X-rays, CT scans, and MRIs, to detect subtle abnormalities that human observers might miss.
- Pathology and histology
AI can analyze digital pathology slides to identify cancerous cells, classify tumors, and predict disease progression. This can improve the accuracy and efficiency of pathology workflows, reducing the burden on pathologists.
- Genomic and proteomic analysis
AI algorithms can analyze genomic and proteomic data to identify disease-related biomarkers and genetic mutations. This can lead to earlier and more accurate diagnoses of genetic disorders and other diseases.
- Analysis of electronic health records (EHRs)
AI can analyze EHRs to identify patterns and risk factors associated with various diseases. This can aid in the early detection of diseases and the identification of patients who are at risk.
AI in disease prediction
AI use in disease prediction is an impressive use case. It enables proactive healthcare by forecasting disease risks and outcomes.
Here are some ways AI is used in disease prediction:
- Risk stratification
AI models can analyze patient data, including genetic, clinical, and lifestyle factors, to predict the risk of developing specific diseases. This allows for early intervention and preventive measures, potentially reducing the incidence and severity of diseases.
- Disease progression prediction
AI can predict the progression of chronic diseases, such as Alzheimer’s disease and Parkinson’s disease, enabling personalized treatment plans and better management of patient care.
AI can predict patient response to specific treatments, helping doctors decide on the best action.
- Epidemiological modeling
AI can analyze environmental data, model the spread of infectious diseases, predict disease outbreaks, and inform public health interventions.
AI in personalized medicine
Personalized medicine is one of the most important AI use cases in life sciences.
Medical professionals aim to tailor treatments to individual patients based on their unique characteristics. AI is a key enabler of this approach in the following ways:
- Genomic profiling and targeted therapies
AI can analyze a patient’s genetic profile to identify specific mutations that drive their disease. This allows for the selection of targeted therapies that are most likely to be effective for that individual.
- Pharmacogenomics
AI can predict how patients will respond to different medications based on their genetic makeup. This can help optimize drug selection and dosing, reducing the risk of adverse drug reactions.
- Personalized treatment plans
AI can integrate data from various sources, including genomics, proteomics, clinical data, and lifestyle factors, to develop personalized treatment plans.
These plans can be tailored to the patient’s needs and preferences, leading to better outcomes.
AI for genomics & biotechnology
Genomics and biotechnology are data-rich fields, making them prime territory for AI’s analytical power. AI is accelerating discoveries and streamlining processes in several ways:
- Genome sequencing and analysis
AI algorithms can analyze vast genomic datasets, identifying patterns, variations, and mutations associated with diseases.
Deep learning models can improve the accuracy and speed of genome sequencing, enabling faster and more comprehensive genomic studies. AI can predict protein folding, a crucial step in understanding protein function and designing new protein-based therapies.
An example is AlphaFold.
- Gene editing and CRISPR technology
AI can optimize CRISPR-Cas9 guide RNA design, improving the precision and efficiency of gene editing.
Machine learning can predict off-target effects of CRISPR editing, minimizing unintended genetic modifications. It can also analyze large datasets to identify potential gene targets for therapeutic intervention.
- Synthetic biology
AI can design and optimize synthetic biological systems, such as engineered bacteria and viruses, for various applications, including drug delivery and bioremediation.
Generative AI can create novel protein sequences and structures with desired functions. It can also aid in optimizing bioprocesses, increasing the yield and efficiency of bio-based production.
AI in clinical trials & research optimization
Clinical trials are essential for evaluating the safety and efficacy of new treatments. AI is optimizing these trials in numerous ways, such as:
- Patient recruitment and selection
AI can analyze electronic health records (EHRs) and other patient data to identify eligible participants for clinical trials, accelerating recruitment.
Machine learning models can predict a patient’s response to treatments, allowing for more targeted and efficient trial design.
- Data management and analysis
AI can automate the processing and analysis of clinical trial data, reducing errors and improving efficiency.
Natural language processing (NLP) can be used to extract relevant information from clinical trial reports and publications.
AI can identify patterns and insights in clinical trial data that traditional statistical methods might miss.
- Adverse event prediction and monitoring
AI can analyze patient data to predict the risk of adverse events during clinical trials, improving patient safety.
- Optimizing trial design
AI can simulate various trial designs to optimize parameters such as sample size, patient population, and treatment duration. This can lead to more efficient and cost-effective clinical trials.
- Remote patient monitoring
AI-powered wearable devices and remote monitoring platforms can gather patient data in real time and transmit it to researchers, allowing for more frequent and accurate data gathering.
AI in content creation
AI is also increasingly important in creating content for the life sciences.
Advanced AI-driven tools like MACg help hasten the writing process, simplify research article summarization, and reduce the time it takes to review hundreds of pieces of literature.
Here are ways that AI is being used in content creation for life sciences:
- Scientific literature summarization
NLP algorithms can summarize complex scientific papers and reports, making it easier for researchers to stay updated with the latest findings. AI can extract key information from scientific publications, such as study designs, results, and conclusions.
- Patient education materials
Generative AI can create patient-friendly educational materials, such as brochures, websites, and videos, that explain complex medical concepts in simple terms.
- Regulatory document generation
AI can assist in generating regulatory documents, such as clinical trial reports and drug approval applications, streamlining the regulatory process.
- Medical writing and communication
AI can assist in writing medical articles, presentations, and other communication materials. It can also help create marketing materials for pharmaceutical and biotech companies.
Conclusion
AI use cases in life sciences are a snippet of how artificial intelligence is revolutionizing healthcare as we know it. It is actively speeding up research, personalizing therapies, and improving patient care. It is no longer merely a futuristic idea.
AI’s contribution to biotechnology and healthcare will only increase as AI technology develops, resulting in more life-improving breakthroughs.
AI in the biological sciences has a promising future, but staying informed is important.
Try out AINGEN’s advanced AI-powered writing and research tool, MACg, today!
READ ALSO: Essential AI Applications Advancing Life Sciences
Start creating & editing content in minutes with AINGENS MACg.
Discover all the amazing things you'll create with AI.
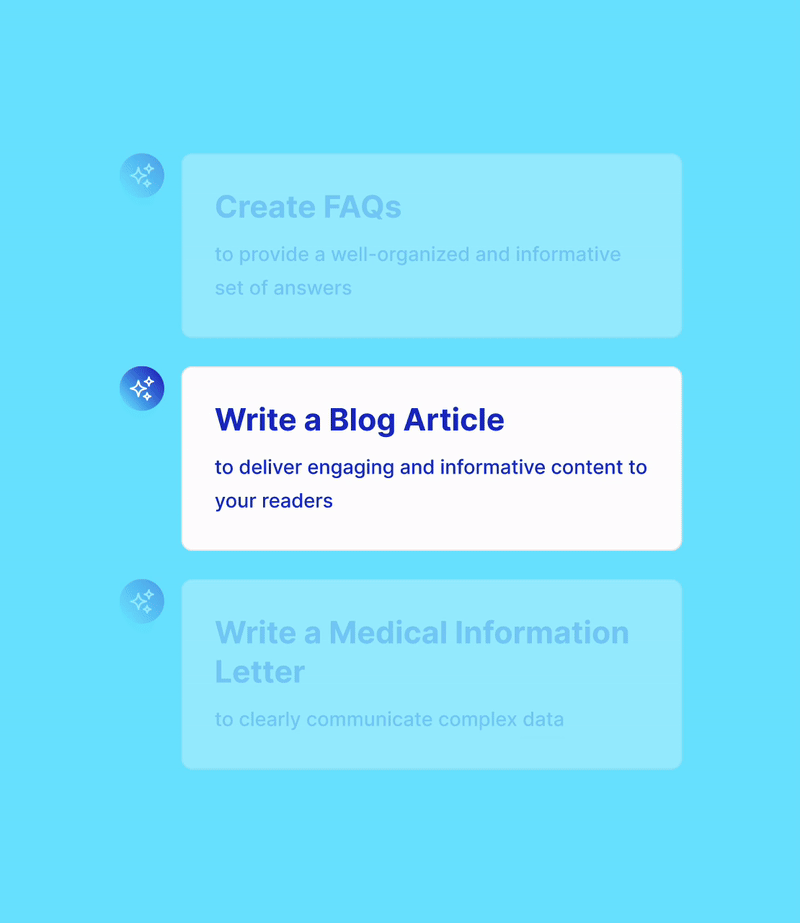