How to Avoid AI Hallucinations in Life Sciences Workflows
Artificial intelligence (AI) is revolutionizing life sciences by enhancing research, content creation, diagnostics, and drug development. However, AI hallucinations—factually incorrect outputs unsupported by data—pose significant challenges. These errors can stem from incomplete training data, complex models, or ambiguous prompts. For instance, AI might fabricate citations or misinterpret findings in biomedical research. An editorial in Nature highlights these issues, emphasizing the need for caution in AI's scientific applications. To mitigate hallucinations, experts recommend refining prompts, employing human oversight, and leveraging Explainable AI (XAI) to ensure transparency and reliability. Regularly updating models and using robust training data can further reduce errors. Despite these challenges, AI's potential in life sciences is immense. By adopting best practices, professionals can harness AI's transformative power while maintaining accuracy and trustworthiness.
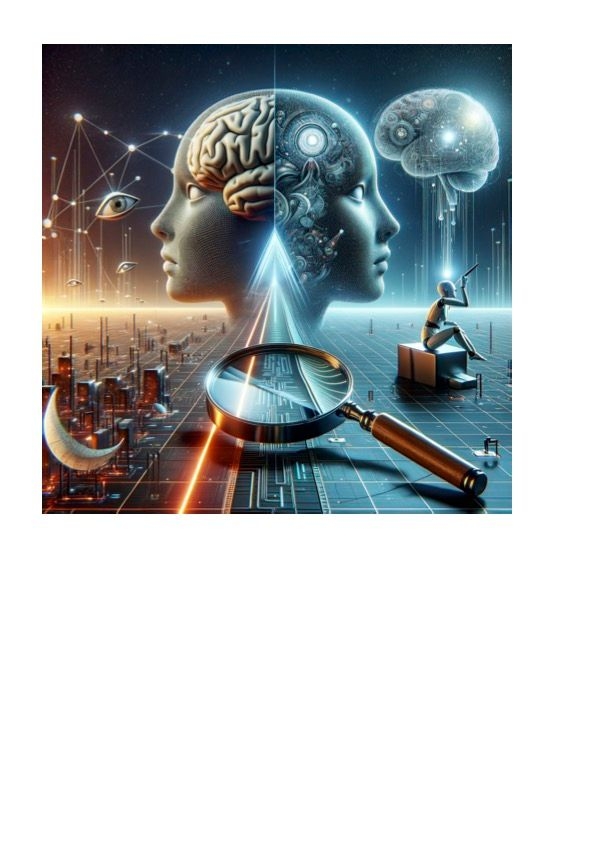
Artificial intelligence (AI) has become a cornerstone of innovation in the life sciences, accelerating research, diagnostics, and drug development. Yet, like any powerful tool, AI is not without its challenges. One of the most pressing issues is that AI hallucinations can create misleading or incorrect outputs. The good news? With a clear understanding and proactive strategies, these risks can be minimized, ensuring AI remains a reliable partner in advancing science.
What Are AI Hallucinations?
AI hallucinations occur when models produce factually incorrect outputs that are unsupported by data or entirely fabricated. These errors can arise from several factors, including incomplete training data, overly complex models, or ambiguous prompts. For example, an AI tool summarizing biomedical research might fabricate a citation or misinterpret statistical findings.
In July 2023, Nature (Schizophrenia) published an article entitled: “ChatGPT: these are not hallucinations – they’re fabrications and falsifications.” This editorial by Robin Emsley critiques ChatGPT's use in scientific writing, focusing on its tendency to fabricate references and falsify information. Emsley shares personal experiences where the AI generated plausible but fictitious citations, reflecting broader research that found high inaccuracy rates in ChatGPT-generated references. The editorial argues these errors are fabrications, not hallucinations, caused by the AI's probabilistic modeling. Emsley warns against the unchecked use of such AI tools in research, highlighting their potential to undermine scientific integrity.
Factors Increasing the Likelihood of Hallucinations
Certain conditions exacerbate the risk of hallucinations:
Ambiguous or Poorly Defined Prompts: Vague inputs can lead AI to generate incorrect outputs.
Complex or Incomplete Training Data: Models trained on biased or insufficient datasets are more prone to errors.
Complex Tasks: Tasks requiring knowledge beyond the model’s training data significantly increase hallucination risks.
Practical Tips for Reducing AI Hallucinations
Refine Your Prompts: Use clear, context-rich prompts to guide the model.
-Example: Instead of “Summarize this research,” ask, “Summarize the diagnostic criteria for rheumatoid arthritis in women aged 30–50, citing recent guidelines.”
Validate Outputs with Human Oversight: Employ domain experts to review AI-generated results, especially for high-stakes applications like clinical trial designs or drug discovery.
Leverage Explainable AI (XAI): Explainable AI (XAI) tools are designed to make AI systems' decision-making processes more transparent and understandable. In life sciences, XAI helps professionals see the reasoning behind AI outputs, such as why a particular protein structure was predicted or how a specific drug candidate was identified.
By providing clear explanations, XAI ensures that AI-generated results can be traced back to their data sources and methodologies, making it easier to verify their accuracy and reliability. This is particularly valuable for regulatory compliance, ethical decision-making, and building trust in AI-assisted workflows.
Incorporate Feedback Loops: Regularly update and retrain models based on real-world performance and errors identified by users.
Utilize Robust Training Data and Curated Knowledge Sources: Curate diverse, high-quality datasets to reduce biases and improve model accuracy. Provide your data or curated references for AI to use for content creation.
Deploy Error Detection Mechanisms:
Implement systems that flag uncertainties or inconsistencies in AI outputs for further review.
AI Hallucinations Should Not Prevent AI Adoption
While hallucinations are a known issue, they are not insurmountable. Combining clear prompt strategies with human oversight and cutting-edge AI tools makes it possible to reduce risks significantly. For example, researchers have successfully implemented these methods to improve AI reliability in predicting protein structures and analyzing clinical data.
As AI reshapes life sciences workflows, its benefits far outweigh the risks when used responsibly. By adopting best practices, professionals can harness AI’s transformative potential while ensuring outputs remain accurate and trustworthy. In a rapidly evolving field, staying informed and vigilant is key to unlocking AI’s full capabilities.
Start creating & editing content in minutes with AINGENS MACg.
Discover all the amazing things you'll create with AI.
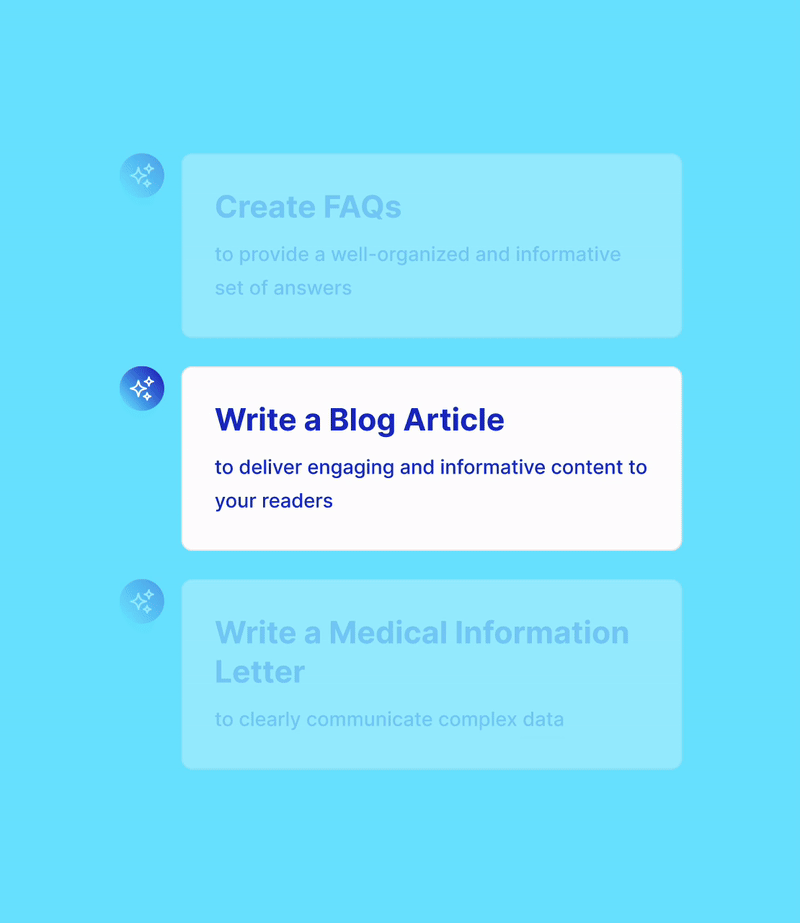