10 AI Terms Life Sciences Professionals Should Know
Artificial Intelligence (AI) is revolutionizing the life sciences, offering groundbreaking advancements in areas like drug discovery and personalized medicine. As AI continues to evolve, understanding key concepts is crucial for professionals aiming to harness its full potential. This article introduces ten essential AI terms, providing a foundation for navigating the rapidly changing landscape of life sciences innovation. From Machine Learning to Explainable AI, these terms will equip you with the knowledge needed to stay ahead in this transformative field.
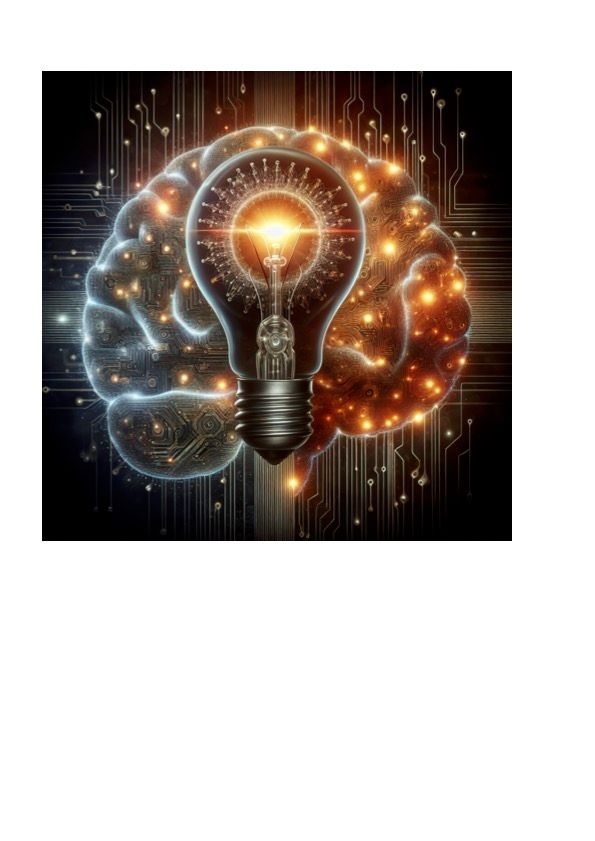
Let’s face it—AI is everywhere, and it’s making waves faster than ever in the life sciences. From predicting protein structures to streamlining clinical trials, AI is shaking up the game. But with all the jargon, it can be tough to keep up. That’s where we come in. The team at AINGENS will bring you ten new terms every week to ensure you stay ahead in this rapidly evolving field. This week’s list breaks down the essentials, giving you the knowledge you need to make sense of the buzz and put it to good use.
Artificial Intelligence (AI)
Why It Matters: AI mimics human intelligence to process information, solve problems, and make decisions. It’s the backbone of tools transforming workflows, accelerating research, and uncovering patterns in massive datasets.
Life Sciences Connection: AI drives smarter solutions for applications like drug discovery, patient diagnostics, and personalized medicine, where complex data demands rapid and accurate processing.
Machine Learning (ML)
Why It Matters: ML, a subset of AI, enables systems to learn from data and improve over time without explicit programming. It’s the engine behind AI’s adaptability and precision.
Life Sciences Connection: ML is revolutionizing drug development by analyzing datasets to predict the success of compounds before trials begin, saving time and resources.
Neural Networks
Why It Matters: Inspired by the human brain, neural networks are systems of interconnected algorithms that excel at pattern recognition and problem-solving. Life Sciences Connection: Neural networks power groundbreaking tools like AlphaFold, which predicts protein structures, aiding researchers in understanding diseases and designing treatments.
Natural Language Processing (NLP)
Why It Matters: NLP allows AI to understand, interpret, and generate human language, making it an essential tool for text-heavy tasks.
Life Sciences Connection: NLP-powered platforms like MACg sift through PubMed articles, summarize findings, and generate clinical documents, enabling professionals to focus on strategic decision-making.
Generative AI
Why It Matters: Generative AI doesn’t just analyze—it creates. From drafting patient education materials to analyzing data, it’s expanding the boundaries of innovation.
Life Sciences Connection: In research, generative AI accelerates the creation of clinical study reports and publications.
Deep Learning
Why It Matters: A sophisticated subset of ML, deep learning uses multi-layered neural networks to uncover patterns in vast amounts of data.
Life Sciences Connection: Deep learning drives diagnostic tools that analyze imaging data, such as X-rays or MRIs, identifying diseases with a precision that often rivals or exceeds human experts.
Explainable AI (XAI)
Why It Matters: Unlike traditional “black box” AI systems, XAI provides transparency, making it easier to understand how conclusions are reached.
Life Sciences Connection: Regulatory professionals rely on XAI to ensure that AI outputs meet compliance standards, are free of bias, and are ethically sound. This builds trust in AI systems used in sensitive areas like patient care.
Reinforcement Learning
Why It Matters: This AI training method relies on trial and error to optimize decisions and improve outcomes, making it invaluable for solving complex problems.
Life Sciences Connection: Reinforcement learning helps refine clinical trial designs, reducing costs and improving outcomes by identifying the most effective protocols.
Overfitting
Why It Matters: Overfitting occurs when an AI model learns too specifically from training data, making it unreliable when faced with new inputs.
Life Sciences Connection: In bioinformatics, avoiding overfitting ensures that AI models generalize well, providing accurate insights across diverse datasets without being biased by specific conditions.
AI Hallucinations
Why It Matters: Hallucinations happen when AI generates confident but incorrect outputs. In life sciences, this risk can have serious consequences.
Life Sciences Connection: Hallucinations can lead to flawed research conclusions or patient safety issues. Ensuring human oversight and rigorous validation of AI-generated findings mitigates these risks.
Integrating AI into the life sciences isn’t just a trend; it’s the beginning of a revolutionary chapter in human discovery. Understanding these foundational terms is the first step in unlocking the incredible potential of AI. By staying curious, informed, and adaptable, life sciences professionals can lead the charge into a future where science and technology work together to achieve breakthroughs we once thought impossible.
Start creating & editing content in minutes with AINGENS MACg.
Discover all the amazing things you'll create with AI.
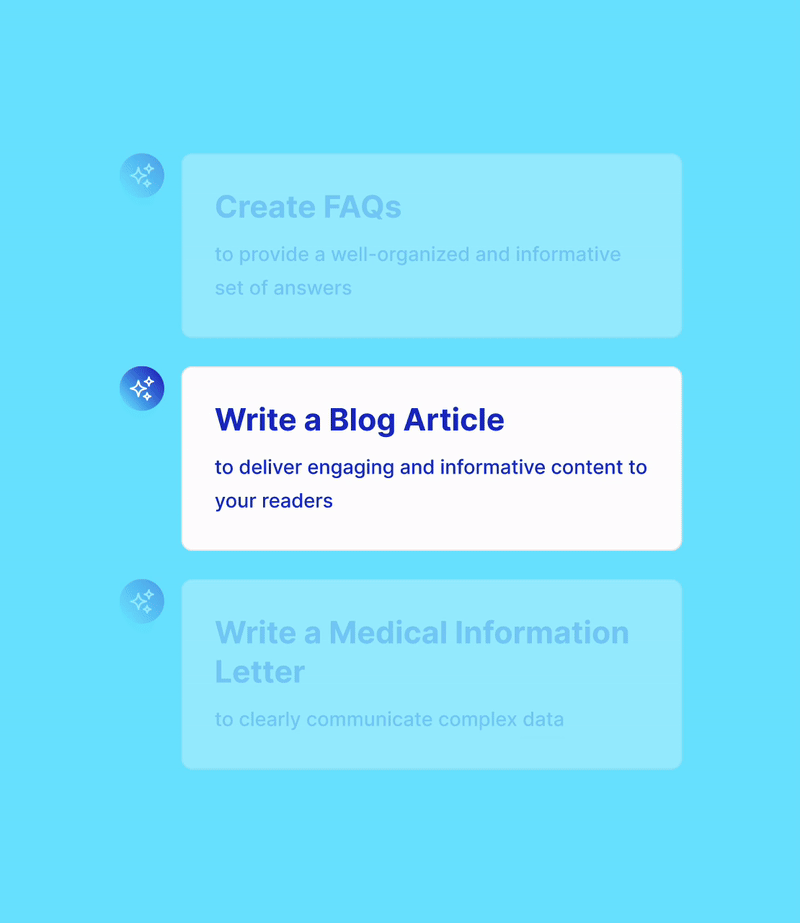